Creating a more accurate tool to forecast the rental demand in the future
To make up for the deficiencies of ‘estimate’, WeYield is trying to use the methods of machine learning to realize forecasting. Discover our new forecasting method right here!
The first step of making the best strategy is to accurately grasp the future market demand changes. In the field of car rental, the demand changes are complex, multifactorial influenced but also potentially regular. WeYield is always committed to providing customers with the most comprehensive information and analysis. Therefore forecasting future demands is a huge challenge to face and overcome.
What did we have before?
Before forecasting, WeYield provided an ‘estimate’ which was based on the history of last year. However, it is not enough. Too much weight of last year will introduce inaccuracies and can not predict this year’s new situation very well.
New forecasting method
To make up for the deficiencies of ‘estimate’, WeYield is trying to use the methods of machine learning to realize forecasting, this will:
- take all historical data into account
- find out correlations among the features, like dates, weekdays, holidays, etc
- put more weight on recent trends
- integrate multiple methods and choose the best algorithm for each client, based on its own data
- provide results on several levels (stations, car, brand) to meet customers needs as much as possible
How do we realize the forecasting?
This is a time series problem. We started from a series of data of continuous dates and rental quantities. We used the data from the previous month as the test set and all the historical data before last month as a training set. In order to improve performance, we removed also some outliers, like a reservation ordered half year before the check-out date. To avoid a relatively large forecasting gap for the coming week, we took into consideration the current number of reservations for future days. We can forecast increments based on the current data instead of on-rent value directly, typically there will not be a large number of new reservations (increments) in a short time.
Then we tried a lot of models to do the regression, like Random Forest, Xgboost. To better extract date characteristics, we added also the information of holidays of different regions in France and weekdays as the features. They perform well when the data is regular and does not have too much noise, unexplained variability within a data sample. In addition, we also attempted some models to involve decomposition into the trend, seasonal, cyclical, and irregular component, like Prophet, a model developed by Facebook in 2017 as well as SArima, which applies an autoregressive model and a moving average model on stationary series.
We fitted the curves with all data on a general level to reduce the noise and then separated forecast results on different stations, cars, brands according to the previous ratio.
Finally, to evaluate the performance, we chose four different indices:
- MSE: Mean Squared Error
- MASE: Mean Absolute Scaled Error
- R2 Score
- MAPE: Mean Absolute Percentage Error
MAPE and R2 are both normalized (between 0 and 1) but have different meanings. MAPE represents how large the differences are between the forecast and ground truth, which would better be close to 0, while R2 shows whether the curve trend is consistent, where 1 is the perfect case. Because of their different evaluation criteria, we also created a score based on these two indices when choosing the model with better performance on a test set to do the forecasting.
What have we achieved now?
Sometimes the estimate and forecast produce almost the same performances because of the similar activities between two years.
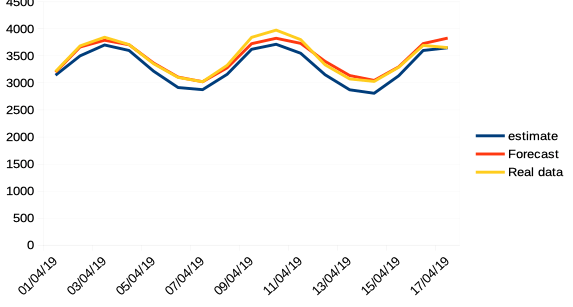
However in most cases, when activities change from year to year. The estimate is no longer able to capture these changes and produce inaccurate values, while the forecast can narrow the gap caused by changes to a certain extent.
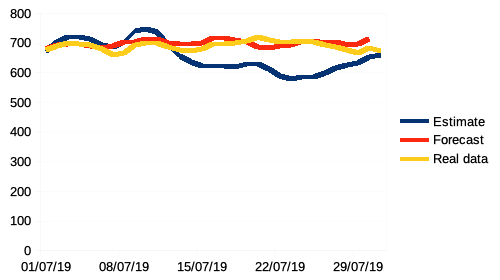
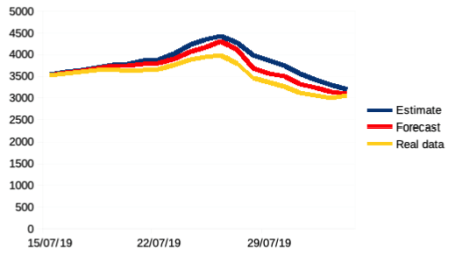
Moving forward WeYield will keep the ‘estimate’ and also provides a new module ‘forecast’ within apps, which contains the forecasting values for 30 coming days as well as 3 past months to observe the performance. This new design is shown as below:

Written by Yue Qiao, Data Scientist